Real-World 5G System
Full-Stack 5G vRAN System for Algorithm Exploration and Over-the-Air Verification of Intelligent, Adaptive, and Machine-Learning-Assisted Baseband Algorithms
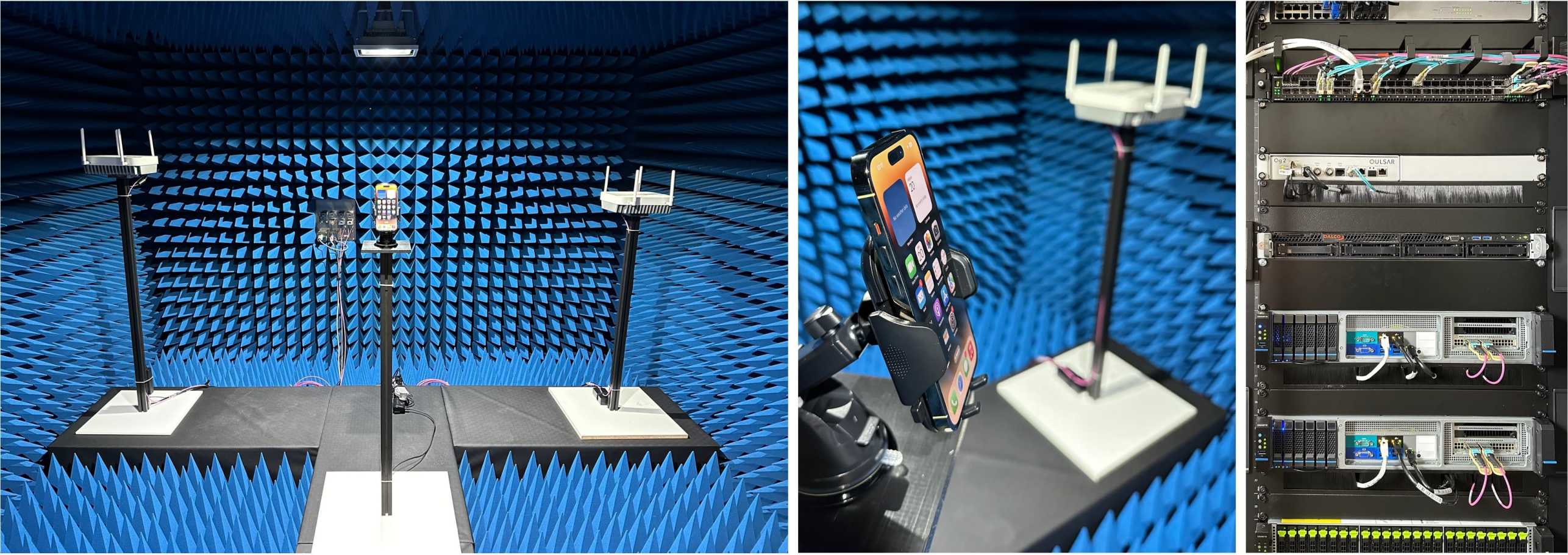
The Integrated Information Processing (IIP) Group at ETH Zurich successfully deployed their 5G virtual radio access network (vRAN) system, which builds upon the external page NVIDIA® Aerial Research Cloud platform. The platform is standards compliant and fully software-defined, which enables rapid prototyping of novel baseband algorithms as well as optimization and verification under real-world conditions. Wireless communications research is typically based on simulations, but only experiments in a real-world 5G system can confirm the efficacy of novel baseband algorithms under realistic operation conditions. Our primary research goals are to use our 5G vRAN system to (i) develop novel, machine learning (ML)-assisted baseband algorithms for future 5G and 6G wireless systems and (ii) optimize and validate our solutions with over-the-air experiments on a real-world system. Furthermore, we will use the 5G vRAN system to continue our work on novel user positioning methods that utilize self-supervised channel charting with channel state information (CSI).
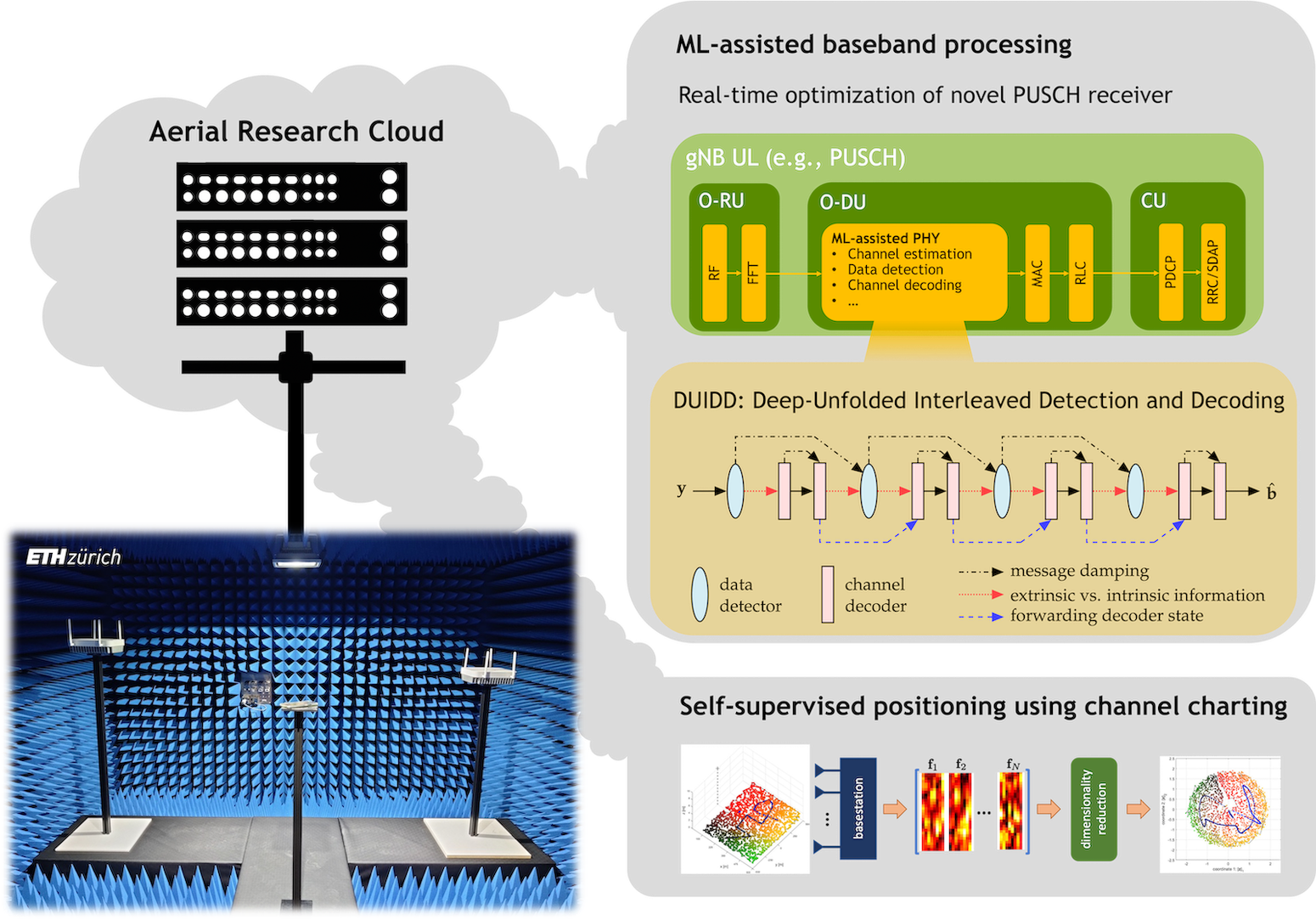
A Software-Defined 5G System Offers the Flexibility of a System Simulator
A software-defined 5G system gives us the capability to implement (in CUDA) novel baseband algorithms that are then executed in real-time. Besides that, we can evaluate the efficacy of our algorithms via over-the-air experiments and under realistic propagation conditions. In contrast to previous real-world prototypes that rely on hardware implementations involving either field-programmable gate array (FPGA) or application-specific integrated circuit (ASIC) implementations, the software-defined nature of the NVIDIA platform reduces development time and verification costs significantly. Furthermore, typical prototypes or commercial access points prevent the extraction of real-time data from the physical layer (e.g., channel state information). With the NVIDIA platform, however, we have the ability to collect labeled data from virtually every part of the signal processing chain. Such datasets are indispensable for the design and training of ML-assisted baseband algorithms and for the development of solutions that are able to adapt to the instantaneous propagation and system conditions.
ML-Assisted Iterative MIMO Detection and Decoding
Recent advances in machine learning (ML) techniques for the physical layer show significant performance gains in various processing stages, including data detection and channel decoding. However, most of these approaches are only trained and verified using system simulations and their efficacy in real systems and under real-world conditions is generally unknown.
Our goal is to implement our recently published external page Deep-Unfolded Interleaved Detection and Decoding (DUIDD) receiver architecture on the NVIDIA Aerial Research Cloud platform. DUIDD fuses MIMO data detection and channel decoding with ML techniques, which was shown in simulations to enable more than 1.4dB performance gains over classical iterative detection and decoding solutions at the same complexity. However, the efficacy of DUIDD under realistic propagation conditions and within a full-fledged 5G system can only be evaluated with real-world measurements that are possible by the NVIDIA platform. Furthermore, over-the-air experiments will also enable us to make DUIDD adaptive to instantaneous system and channel conditions (e.g., properties of the physical radio channel, number of active users, interference, code rate, modulation scheme, etc.), which we expect to offer additional performance and complexity improvements.