Positioning with Wireless Signals and Channel Charting
Localization services for wireless devices play an increasingly important role in our daily lives and a plethora of emerging services and applications already rely on precise position information. Widely used on-device positioning methods, such as the global positioning system (GPS), enable accurate outdoor positioning and provide the users with full control over what services and applications are allowed to access their location information. In order to provide accurate positioning both indoors as well as in cluttered urban scenarios without line-of-sight satellite connectivity, powerful off-device positioning systems have emerged. Such positioning systems work based on information about the propagation conditions between transmitter and receiver (typically a basestation or access point), the so-called channel state information (CSI). The CSI is measured at the infrastructure basestations or access points and processed with deep neural networks in order to obtain accurate positioning information. The IIP Group is working at the frontier of such emerging positioning technologies and has developed state-of-the-art supervised localization pipelines as well as the first self-supervised method called Channel Charting, which enables pseudo-localization without reference position information.
Channel State Information (CSI)-Based Positioning
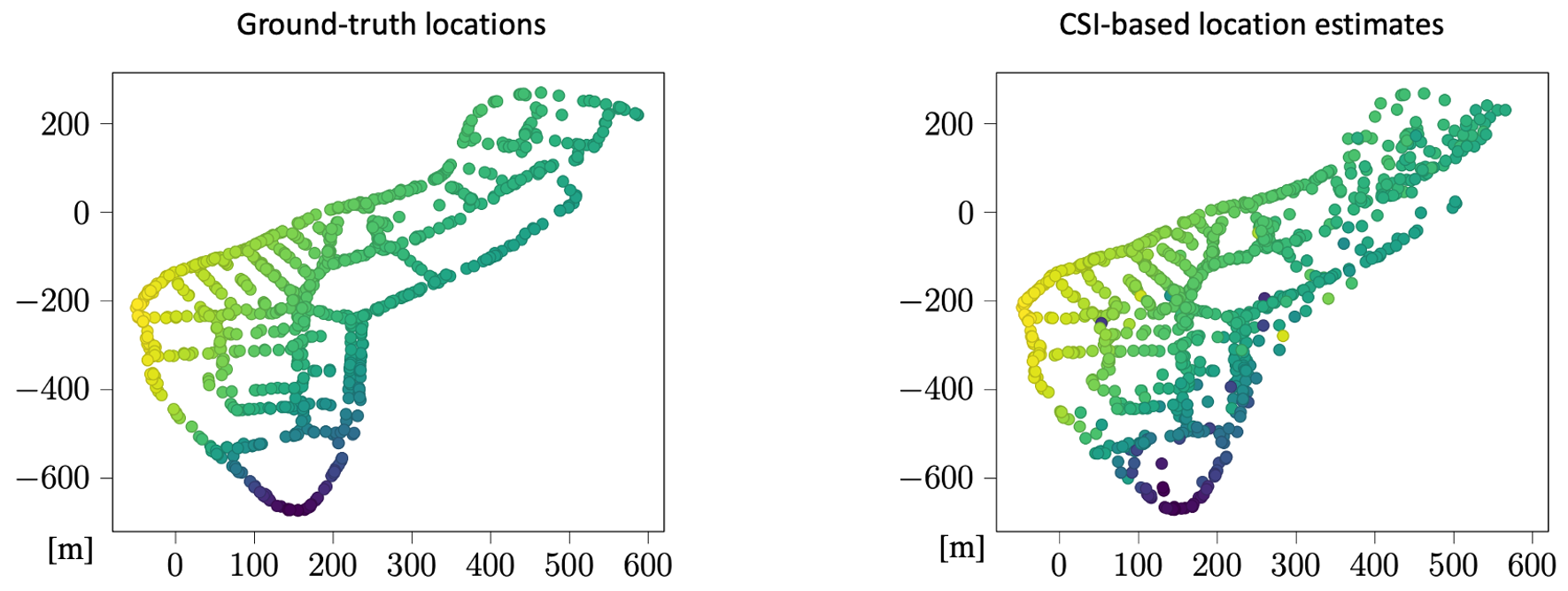
In order to enable reliable data transmission, wireless systems estimate the channel's transfer function (e.g., in the form of an impulse response) using a training sequence that is known to both the transmitter and receiver. The measured CSI contains information about the propagation environment, which can be utilized for wireless positioning. Classical wireless positioning techniques utilize geometrical models for wave propagation in order to perform triangulation or trilateration. Unfortunately, such approaches require accurate calibration and synchronization among the transmitter and receiver. They also require mostly idealistic line-of-sight propagation conditions to all receive antennas, which often prevents accurate positioning indoors or in dense urban scenarios that contain multiple scatterers. More recently, geometry-free wireless positioning systems that process the measured CSI with the aid of DNNs have emerged. Their principle is simple yet effective: Train a DNN with a dataset consisting of CSI measurements and ground-truth locations obtained via a reference positioning system. Then, use the DNN to process new CSI measurements to generate location estimates. Such positioning systems enable accurate localization even under challenging propagation conditions that prevent approaches relying on geometrical models. The IIP Group has shown that meter-level and centimeter-level accuracy can be achieved for real-world outdoor and indoor scenarios with measured CSI, respectively.
Besides CSI-based positioning, the IIP Group is also concerned with privacy and security aspects that arise from such emerging positioning technologies. Since the extraction of CSI is possible by virtually everyone in the vicinity of the transmitter, CSI-based positioning can be done without the transmitter's (e.g., a mobile user) consent. By extracting location estimates from CSI, privacy is at stake. For example, one can learn the users' behavior (e.g., what stores or bars they frequently visit), their socioeconomic status (e.g., based on where they live and work), or even gain sensitive information about their health (e.g., if the user frequently visits a specialized health clinic). One can even envision concrete security threats, as location information enables one to determine when a user is leaving their home, parking their car, or withdrawing money. The IIP Group is developing novel and effective countermeasures that prevent accurate CSI-based off-device localization without sacrificing quality-of-service.
Channel Charting
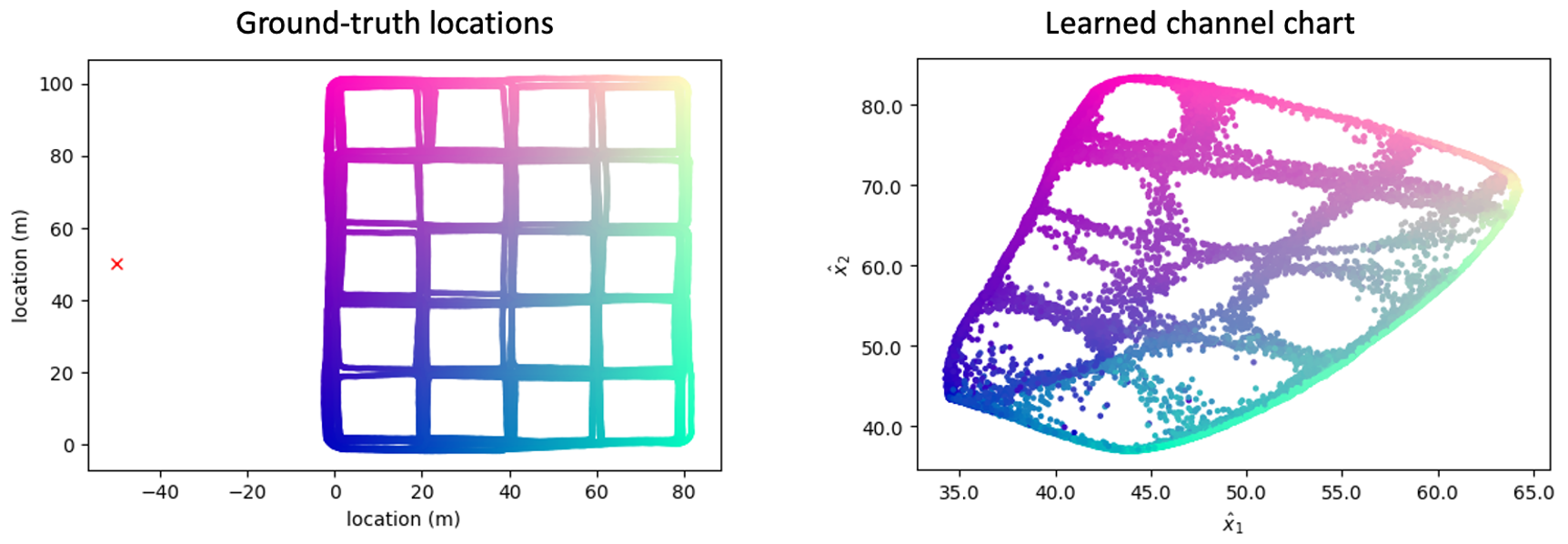
In many practical applications, absolute location information is not necessary and ground-truth location information is unavailable. Channel charting is an emerging technology that learns a mapping from channel state information (CSI) to a so-called channel chart in which nearby datapoints indicate nearness in real space. In other words, the learned channel chart captures the nearby spatial geometry of the transmitting user equipments (UEs), effectively encoding relative (or logical) UE locations. Channel charting is self-supervised as the mapping from CSI to the channel chart is learned only using a database of passively collected CSI measurements. Such a data-driven pseudo-localization approach has the advantages of being scalable and avoiding reference location information, e.g., from global navigation satellite systems (GNSSs). The self-supervised nature of channel charting also avoids the need for line-of-sight (LoS) propagation conditions or (costly) measurement campaigns, while enabling the infrastructure basestations or access points to perform cognitive and predictive radio access network (RAN) tasks which are tied to UE location. Channel charting was invented by Prof. Studer and external page Prof. Olav Tirkonnen from Aalto University and enables location-aware and predictive tasks for situations in which ground-truth location information is unavailable. In case you would like to learn more about channel charting, please visit the external page Channel Charting Resources website.