Paper on attacking and defending deep-learning-based off-device wireless positioning systems now available online!
Our recent arXiv preprint discusses methods to address location privacy issues with off-device positioning systems.
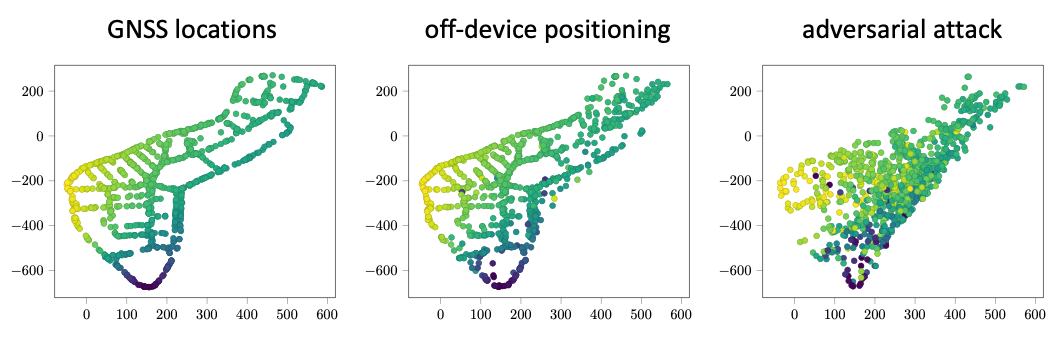
On-device positioning methods, such as global navigation satellite systems (GNSS), provide the users with full control over who can access location information. In contrast, emerging off-device positioning methods that process channel state information enable virtually anyone in a device's range to estimate its location. Does this mean that location privacy is forever lost?
In our recent work, we demonstrate that on-device adversarial attacks significantly reduce the accuracy of ML-based off-device positioning systems without noticeably degrading quality-of-service. We also investigate defenses to counter such attack mechanisms; this leads to an arms race between off-device defenses and on-device attacks. We discuss the limitations of our results and implications on protecting location privacy in future wireless systems.
A preprint of our paper, which is a collaboration between researchers from Cornell University, Ericsson, Nokia Bell-Labs, NVIDIA, and ETH Zurich, is available on external page arXiv.